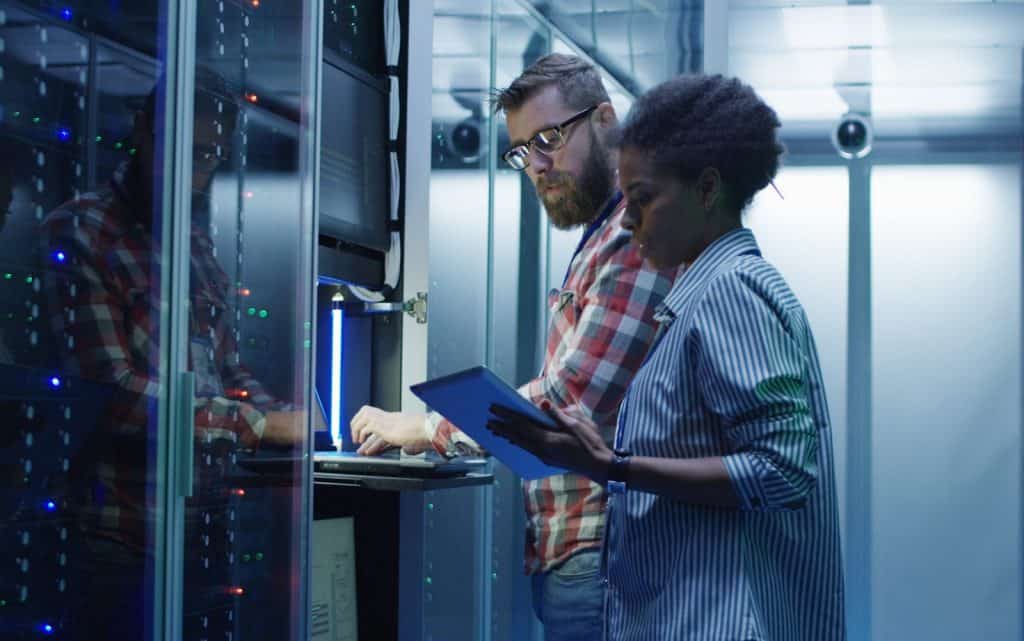
That could make it easier to run machine learning on mobile devices.
Machine learning has given computers the ability to do things like identify faces and read medical scans. But when it’s tasked with interpreting videos and real-world events, the models that make machine learning possible become large and cumbersome. A team from the MIT-IBM Watson Lab believe they have a solution. They’ve come up with a method that reduces the size of video-recognition models, speeds up training and could improve performance on mobile devices.
The trick is in shifting how video recognition models view time. Current models encode the passage of time in a sequence of images, which creates bigger, computationally-intensive models. The MIT-IBM researchers designed a temporal shift module, which gives the model a sense of time passing without explicitly representing it. In tests, the method was able to train the deep-learning, video recognition AI three times faster than existing methods.
The temporal shift module could make it easier to run video recognition models on mobile devices. “Our goal is to make AI accessible to anyone with a low-power device,” said MIT Assistant Professor Song Han. “To do that we need to design efficient AI models that use less energy and can run smoothly on edge devices where so much of AI is moving.”
By reducing the computing power required for training, the method might also help reduce AI’s carbon footprint. It could help platforms like Facebook and YouTube spot violent or terrorist footage, and it might allow medical institutions like hospitals to run AI applications locally, rather than in the cloud, which could keep sensitive data more secure. The researchers will present their findings in a paper at the International Conference on Computer Vision later this month.